Herd Accountability from Auditing Privacy-Preserving Algorithms
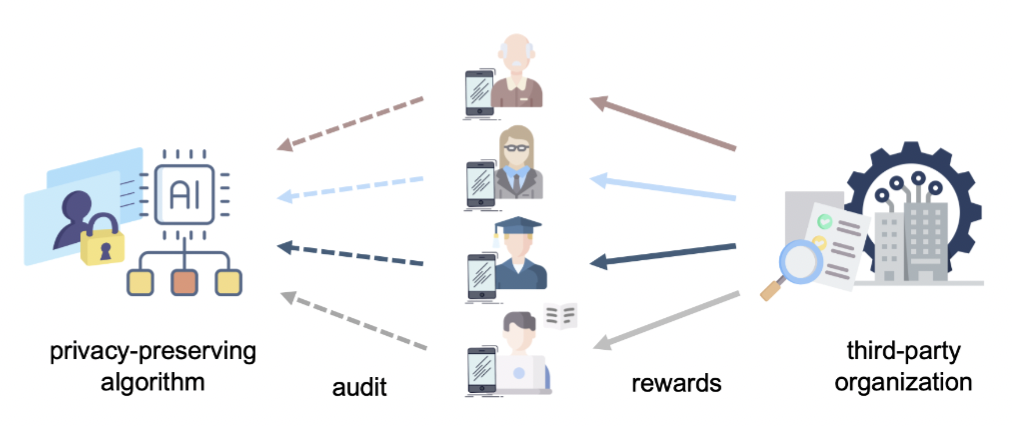
Paper (This prior work is published in Decision and Game Theory for Security (GameSec 2023)): https://link.springer.com/chapter/10.1007/978-3-031-50670-3_18
Motivation
Privacy-preserving AI algorithms are widely adopted in various domains, but the lack of transparency might pose accountability issues. While auditing algorithms can address this issue, machine-based audit approaches are often costly and time-consuming.
Herd audit, on the other hand, offers an alternative solution by harnessing collective intelligence. Nevertheless, the presence of epistemic disparity among auditors, resulting in varying levels of expertise and access to knowledge, may impact audit performance. An effective herd audit will establish a credible accountability threat for algorithm developers, incentivizing them to uphold their claims.
Our approach
We develop a systematic framework that examines the impact of herd audit on algorithm developers using the Stackelberg game approach.
The herd audit framework with the third-party organization providing rewards and penalties. In auditing privacy-preserving algorithms, there is a public-known privacy protection agreement for a budget $\epsilon^\prime$. The developer who is either responsible ($\omega=g$) or negligent ($\omega=b$) takes the first step by selecting the executed privacy budget $\epsilon$ according to his strategy $q(\epsilon|\omega)$. Then, the auditor, characterized by an epistemic factor $\lambda$, determines how to gather information $s$ that leads to her audit confidence $r(\omega|s)$ regarding compliance or non-compliance.
Main Results
- The optimal strategy for auditors emphasizes the importance of easy access to relevant information and the appropriate design of rewards, as they both increase the auditors’ confidence and assurance in the audit process.
- Herd audit serves as a deterrent to negligent behavior of the algorithm developer.
- By enhancing crowd (herd) accountability, herd audit contributes to responsible algorithm development, fostering trust between users and algorithms.
Some related research papers
Rational inattention
- S. Kapsikar, I. Saha, K. Agarwal, V. Kavitha, and Q. Zhu, “Controlling fake news by collective tagging: A branching process analysis,” IEEE Control Systems Letters, vol. 5, no. 6, pp. 2108–2113, 2021.